How Trends in Computer Vision Are Driving the Need for AI Security Cameras
New technologies, such as artificial intelligence (AI) and machine learning, help make sense of what can be “seen” in security video footage.
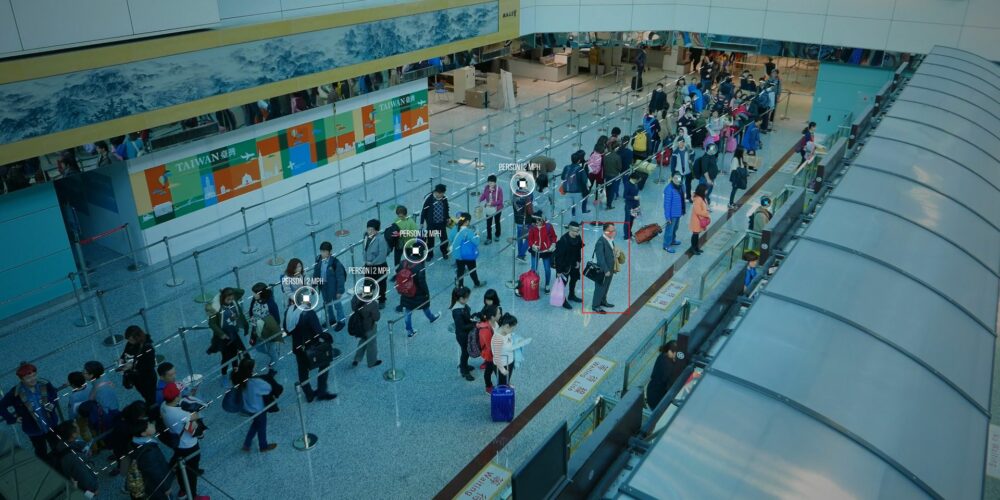
Image courtesy of Hailo
The security market is awash with video.
The prevalence of network connectivity, including wireless to remote locations, plus Moore’s Law-style advances in the power and capability of video cameras, not to mention society’s desire to feel safer everywhere, has led to the adoption of billions of security cameras worldwide, generating thousands of petabytes of video data daily.
What to make of all that video? That’s the challenge. In most cases, security video is stored away (we can thank advances in cloud computing for unlimited storage) until something happens and the footage needs to be checked, whether it’s in a supermarket or in a home.
For more immediate security applications — surveillance, traffic monitoring, an emerging class of “smart city” solutions — video often travels across a network, including through the cloud, to a computer system where it’s analyzed.
It’s there that new technologies, such as artificial intelligence (AI) and machine learning, help make sense of what can be “seen” in all that video.
For modern security applications, the field of computer vision is about developing systems that can identify and understand people and objects in video data — a person in distress at a stadium, a bag left on a train platform, the license plate number of a speeding vehicle. And these days, computer vision is undergoing a significant evolution that will take it from being a specialty application to a near-ubiquitous, always-protecting feature of security systems. The key is smarter cameras.
Trends in Computer Vision
Computer vision is a growing market, influencing everything from augmented reality, to autonomous driving, to smart agriculture. In the security industry, AI-based computer vision promises greater personal safety and more automated, proactive protection of places and infrastructure based on the technology’s ability to analyze video data.
But making sense of all the video generated by installed base of video cameras has its challenges. And for computer vision to pervade security applications, camera solutions need to address several trends:
Higher resolutions. In consumer electronics, high-resolution video is the norm. Even Ring video doorbells have begun to push above Full HD, 1080p resolution. Security cameras are heading in that direction, too, opening the door to new computer vision applications.
All those additional pixels, plus wide-angle lenses, make it possible to better identify people, things, or even behaviors over a larger field of vision. The trick is maintaining that resolution as the video moves from the camera to the analytics system. Often, video must be downscaled to save network bandwidth, which could limit the effectiveness of computer vision.
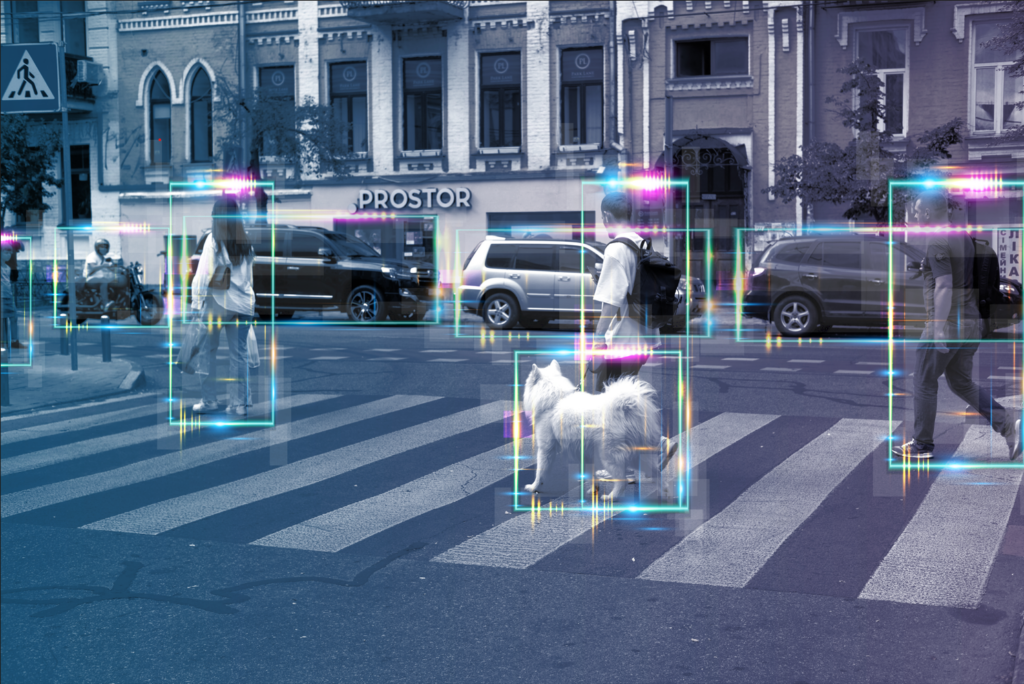
Image courtesy of Hailo
Privacy protection. As video cameras become ubiquitous and their resolution improves, it is becoming easier to identify people in a video feed. This capability is specifically important for re-identification applications, in which the same person is tracked multiple times in the same camera at different time stamps, or over multiple cameras.
This application is being used both for statistical purposes as well as investigation of events and tracking suspicious activity. In these cases, it is becoming increasingly important to address concerns about privacy protection.
This can include automatically blurring faces, a process commonly called anonymization, or processing video feeds locally on the edge and assigning metadata to describe people or things. In other words, rather than simply streaming and storing video of a person in a red shirt, a computer vision system streams and stores only the metadata indicating it perceived a person in a red shirt.
Only if there’s a security need to find all the people wearing a red shirt would the video itself be accessed. However it’s accomplished, privacy protection needs to develop alongside computer vision.
Diversification of analytics. Until recently, computer vision and analytics have been about safety and security — identifying potential threats to people and places. Increasingly, though, organizations want to learn more from their installed base of video cameras.
For example, a retail store may want to identify shoplifters, but it’s also now interested in analyzing the movement of customers through a space to understand, for example, the effectiveness of store design, product placement, or marketing displays.
Smart cities not only embrace video for its security applications, but also for its ability to reveal traffic patterns. In either case, different analytical algorithms may be applied to different video streams of the same scene, requiring a more powerful, efficient computer vision solution.
Emergence of video processing units (VPUs). That more powerful, efficient solution comes in the form of new vision processors. These systems on a chip (SoC) have a lot to handle, including higher resolution video, solutions to protect privacy, and potentially several forms of analytical processing.
There are established technologies for enhancing video through image stabilization, blur reduction, and other techniques. Combine those capabilities with AI-based computer vision processing, and cameras not only “see” better and more clearly, but they also better understand what they see and create a better visual image of the scene.
What’s more, by applying AI to common vision enhancement pipelines, like reducing image “noise” in low light conditions, this new class of VPUs perform faster and more efficiently, even with less available light.
Distributed analytics. To date, these two video processing tasks — video enhancement and video analytics — have happened separately. The camera itself improves the picture, but the improved video gets sent to a cloud system or control center for analysis.
There are a couple of reasons this is inefficient. First is the latency and bandwidth consumption of constantly sending video over a network. Second, and related, is that for many security and other “smart” video applications, action should be taken in real-time, meaning the analysis should take place out where the camera is located. This has meant computer vision analytics is increasingly distributed, not centralized.
Computer Vision at the Edge
In computing terms, these distributed locations are at the edge. In recent years, the success of cloud computing, where applications and processing run in large data centers, has spawned a correlating interest in edge computing, where applications and processing run on devices closer to where they’re needed.
AI especially benefits from an edge model because, in many cases, the data being processed is generated where the AI processing and analytics are needed most. For example, the manufacturing industry uses AI alongside robotics to quickly spot quality issues in a factory. Healthcare facilities use AI in imaging systems to better identify life-threatening conditions and improve patient outcomes.
In security, AI-based computer vision has similar requirements. Depending on the application, as a security camera collects video, it makes sense if the AI and analytics processing are handled closer to the camera, if not in the camera itself.
This allows quicker action, in some cases prompted by AI itself. It also requires new processing technology, including chips that are designed to handle the kind of neural networks used in AI.
Forcing neural networks, which handle data in a scattered fashion like the synapses in a brain, through traditional, linear processors requires power and energy that edge devices like cameras can’t afford. Many video cameras are installed in austere environments, away from reliable power sources, so if they’re to use computer vision processing, that processing has to be efficient. It also has to be powerful.
Edge AI Processors for Computer Vision
In light of advances in computer vision and demand for AI analytics at the edge of a security system — where events happen — the industry has developed specialized edge AI processors. These processors can be integrated into smart cameras or into aggregators located at the edge, such as video management systems (VMS) or network video recorders (NVR) that take streams from existing video cameras and apply AI algorithms to that video.
Edge AI can be a powerful enabler in two key ways. The first is by detecting people, events, or situations through various analytics and automatically triggering an alert or response. The second is by automatically analyzing video and applying metadata so it can be more easily searched later.
For example, instead of combing through stored video for someone in a red shirt, the security professional can search for “red shirt” and be presented with all relevant footage.
Granted, replacing a billion security cameras worldwide with smart cameras that include edge AI processors would be a tall order. But the ultimate goal is to push as much computer vision processing as possible — both AI and video enhancement processing — to the camera edge, where it can do the most good.
Whether that’s smart cameras or cameras made smarter by attaching them to a system with vision processors, the security industry is in a better position than it’s even been to exploit AI for the betterment of all.
Yaniv Iarovici is vice president of business development for Hailo.
If you enjoyed this article and want to receive more valuable industry content like this, click here to sign up for our FREE digital newsletters!
Security Is Our Business, Too
For professionals who recommend, buy and install all types of electronic security equipment, a free subscription to Commercial Integrator + Security Sales & Integration is like having a consultant on call. You’ll find an ideal balance of technology and business coverage, with installation tips and techniques for products and updates on how to add to your bottom line.
A FREE subscription to the top resource for security and integration industry will prove to be invaluable.